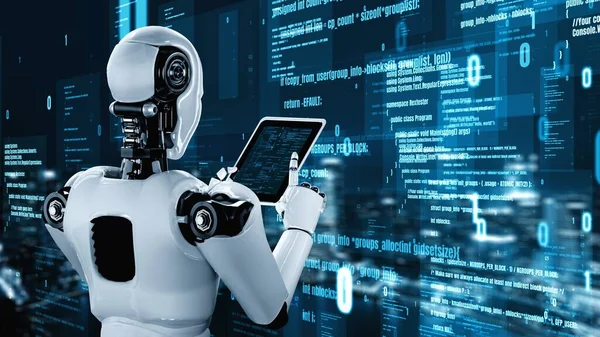
In the quickly developing scene of innovation, two fields have arisen as the main impetuses behind advancement and change: artificial reasoning (computer-based intelligence) and information science. This dynamic duo has revolutionized business operations, offering unprecedented insights, automation, and decision-making capabilities. This article will explore the synergies between tech, artificial intelligence, and data science, their contributions, and their impact on various industries.
Understanding Artificial Intelligence
Manufactured reasoning, frequently called artificial intelligence, alludes to creating PC frameworks that can perform undertakings that commonly require human knowledge. These errands incorporate critical thinking, discourse acknowledgment, learning, and direction.
Computer-based intelligence can be grouped into two primary classes: restricted computer-based intelligence, intended for a particular work, and general artificial intelligence, which can comprehend, learn, and apply information across different spaces.
Machine Learning and AI
Tech, artificial intelligence, and data science are subsets of computer-based intelligence that form frameworks to gain from information. Through ML calculations, PCs can recognize designs, make forecasts, and persistently further develop execution without express programming. This capability has paved the way for natural language processing, image recognition, and recommendation systems advancements.
Deep Learning
Profound Learning (DL) is a particular area of ML enlivened by the design and capability of the human cerebrum’s brain organizations. Deep brain networks with numerous layers (thus the expression “profound”) succeed in taking care of enormous volumes of unstructured information. This has proven invaluable in tasks such as image and speech recognition, language translation, and games like Go.
The Job of Information Science
Information science is an interdisciplinary field that utilizes lucid systems, cycles, assessments, and designs to separate experiences and information from composed and unstructured information. Its methods include genuine examination, artificial intelligence, data mining, and broad data assessment.
Data Collection and processing
The foundation of data science lies in data, and the quality of insights derived depends on the data’s accuracy and relevance. Data scientists are tasked with collecting and preprocessing vast amounts of data to ensure it is suitable for analysis. This involves cleaning, transforming, and organizing the data into an effectively utilized format.
Exploratory Data Analysis
EDA is a vital stage in information science where experts investigate and imagine information to distinguish examples, patterns, and connections. Information researchers gain a more profound comprehension of the information through different factual and graphical strategies, illuminating the resulting demonstration and examination steps.
Predictive Modeling
Predictive modeling is at the heart of data science, allowing organizations to forecast future trends and outcomes. Calculations play a significant role in prescient display, empowering the improvement of models that can make exact forecasts given verifiable information.
The Symbiosis of AI and Data Science
The symbiosis of artificial intelligence (AI) and data science forms a dynamic partnership, driving innovation and transforming industries. Together, they create a continuous improvement cycle, optimizing processes across healthcare, finance, retail, and beyond.
This synergy enhances efficiency and raises ethical considerations, urging a careful balance between technological progress and societal well-being. The future promises even greater integration, with trends like edge computing and explainable AI shaping a brighter tomorrow.
Data-Driven AI
AI systems are only as effective as the data they are trained on. Data science supplies high-quality, relevant data to train AI models. The symbiotic relationship ensures AI adapts to real-world scenarios, enhancing accuracy. Data-driven AI relies on quality data, improving decision-making across applications and industries.
Continuous Learning
The iterative nature of both AI and data science fosters continuous learning and improvement. As AI systems process new data, they adapt and enhance their performance. Data scientists, in turn, use the feedback and outcomes from AI applications to refine models, ensuring a cyclical optimization process.
Automation and Efficiency
Data science-driven AI brings previously unheard-of levels of efficiency and automation to various businesses. Tasks requiring extensive manual effort can now be streamlined through AI-driven processes, allowing organizations to allocate resources strategically and focus on high-value activities.
Impact on Industries
The impact of artificial intelligence (AI) and data science on industries is transformative. In healthcare, predictive diagnostics and personalized treatment plans improve patient outcomes—finance benefits from AI-driven fraud detection, risk management, and algorithmic trading. Retail sees optimization through inventory management and customized customer experiences.
These technologies streamline operations, enhance decision-making, and forecast trends, creating a ripple effect of efficiency. As AI and data science evolve, their influence across sectors highlights a paradigm shift in how businesses operate and innovate, setting the stage for a technologically empowered future.
Healthcare
The marriage of AI and data science has revolutionized healthcare by enabling predictive diagnostics, personalized treatment plans, and drug discovery calculations that can dissect patient information to recognize potential chances for well-being. At the same time, information-driven experiences support proof-based decision-making for medical service suppliers.
Finance
In the financial sector, tech, artificial intelligence, and data science are harnessed for fraud detection, risk management, and algorithmic trading. Predictive modeling assists in forecasting market trends, while automated systems streamline routine financial tasks, enhancing overall operational efficiency.
Retail
Retailers leverage technology, artificial intelligence, and data science to optimize inventory management, personalize customer experiences, and forecast demand. Recommendation engines, powered by machine learning, analyze customer behavior to suggest products, increasing customer satisfaction and driving sales.
Ethical Considerations
As artificial intelligence and information science become fundamental pieces of our day-to-day routines, moral contemplations should be at the forefront of their turn of events and organization. Issues like predisposition in calculations, information security, and responsibility require cautious regard to guaranteeing these advancements benefit society.
Future Trends
Future trends in technology signal a paradigm shift, with the integration of artificial intelligence (AI) and data science at the forefront. Edge computing emerges, minimizing latency and enabling real-time decision-making for applications like autonomous vehicles.
Explainable AI gains prominence, addressing concerns about transparency and accountability in algorithmic decision-making. These trends signify a more interconnected, efficient, and understandable technological landscape. As AI and data science evolve, their convergence with emerging technologies promises to redefine the possibilities and capabilities of the digital age.
Edge Computing
Integrating AI and data science with edge computing is poised to be a game-changer. Edge figuring permits information handling nearer to the source, diminishing inactivity and empowering ongoing independent direction.
This is especially significant in applications like autonomous vehicles and IoT gadgets. Edge computing revolutionizes data processing by decentralizing it, bringing computation closer to the data source.
This minimizes latency and enables real-time decision-making, making it pivotal for applications like IoT devices and autonomous vehicles. The shift towards edge computing signifies a transformative approach to data management in the ever-evolving technological landscape.
Explainable AI
The black-box nature of some tech-based artificial intelligence science models has raised concerns about transparency and accountability. Reasonable simulated plans to resolve this issue by making simulated calculations more interpretable; it is ventured to give experiences into how choices.
Here, trust and understanding are the principals. AI focuses on making artificial intelligence algorithms interpretable, promoting transparency and understanding of decision-making processes for improved accountability and trust.
Conclusion
The combination of computerized reasoning and information science has introduced another time of mechanical progression, changing how we work, live, and interface. As these fields continue to evolve, the symbiotic relationship between them will play a pivotal role in shaping the future of innovation. By harnessing the power of data-driven AI, we can unlock unprecedented possibilities and address complex challenges, paving the way for a brighter, more efficient, and ethically responsible technological landscape.
2.How does Data Science contribute to Artificial Intelligence?
Data Science provides the essential foundation by collecting, preprocessing, and refining data, which is crucial for training AI models. It ensures that AI systems learn from high-quality, relevant information.
3.What industries benefit most from the integration of AI and Data Science?
Healthcare, finance, and retail are among the industries benefiting significantly. AI and Data Science enhance diagnostics, optimize financial processes, and personalize customer experiences, respectively.
4.What is Edge Computing in the context of AI and Data Science?
Edge Computing involves processing data closer to the source, reducing latency and enabling real-time decision-making. It's particularly crucial for applications like IoT devices and autonomous vehicles.
5.Why is Explainable AI important?
Explainable AI aims to make the decision-making processes of AI algorithms more transparent and interpretable. This is crucial for ensuring accountability, building trust, and addressing concerns about biased or opaque AI systems.